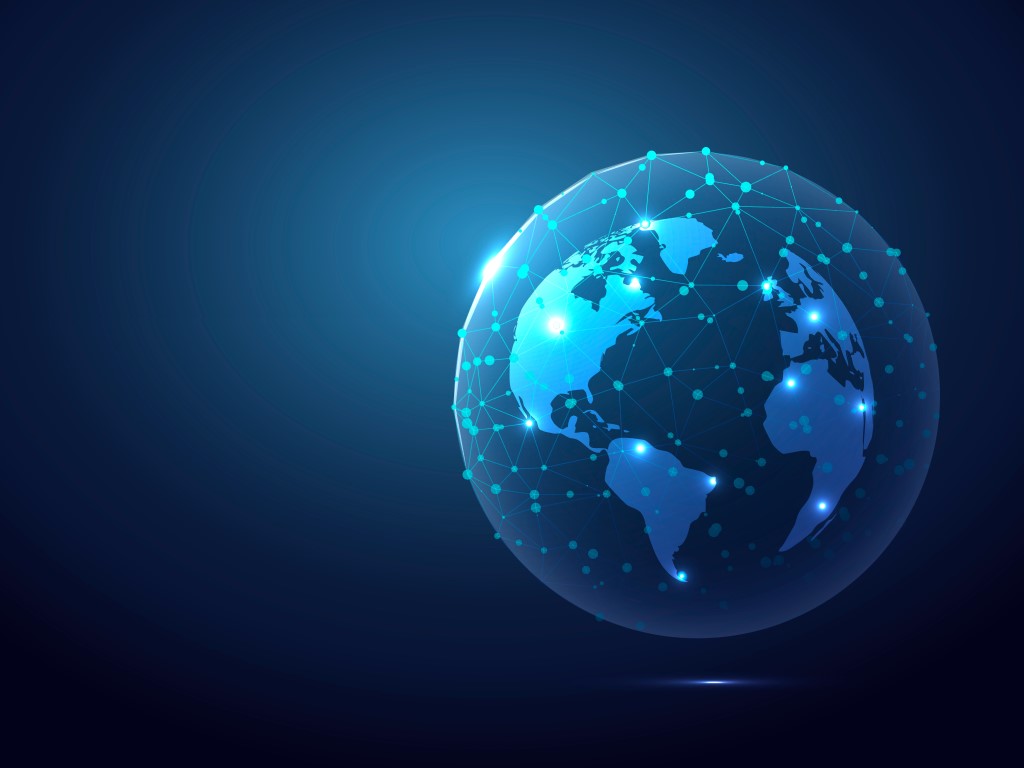
Business and Machine Learning: Key Problems It Can Solve

Machine learning is in its heyday, but the question for many businesses remains: How can it solve the existing problems? In this post, we will look at business areas in which ML could make a good case. Hopefully, it helps you see what can be done so you can figure out how it might be applied to your business.
Where can machine learning be applied?
Machine learning is applied for complex decisions where a lot of factors are at play. That said, problems that suggest a clear chain of steps to follow in order to resolve them aren’t likely to need machine learning at all. The same goes for having existing cases of successfully addressing problems using ML. In these scenarios, applying algorithms may be a redundant step.
On the other hand, here’s a list of common business cases where leveraging machine learning is recommended.
Face recognition
It’s somewhat problematic to come up with a set of rules for the face recognition process, given that there are different nuances like skin and eye color, shapes, and angles that might impede accurateness. Nevertheless, machine learning algorithms can be trained to detect eyes, mouth, nose, etc. Facebook already uses those, and there are also many free face recognition tools.
Spam filters
Email spam filters go by simple rules, for example, they can identify and block compromised domains and IP addresses. However, these algorithms tend to lack in filtering incoming messages based on a user’s personal preferences. That is where applying machine learning can be the right solution.
Recommendation engines
The tastes of each person in music, movies, and products are different. Tech giants like Netflix, Spotify, Amazon rely on machine learning and data to predict what a user might prefer.
Speech recognition
Machine learning makes it possible to translate a spoken language into text. The significant challenges are a variety of accents, loud environments, and the fact that speech recognition algorithms need lots of data. The top companies operating in this area are Nuance Communications, Google LLC, Amazon.com, Inc, Apple, Inc, IBM Corporation, Microsoft Corporation, and Baidu.
Online ads
It’s impossible to predict which Facebook ads will prompt a particular user to click. Machine learning can identify patterns in user behavior and determine which ads resonate with which group of users.
Fraud detection
Little cases of fraud detection can be fended off with a clear-cut set of rules. That is because fraud techniques are continually evolving, so your protection needs to be adapted all the time.
Upselling
Machine learning systems can process data that concerns your buyer profile and types of things they are looking to buy from you. You can tweak the algorithm to target clients that are land concrete pages of your website. For example, if you’re selling phones, you can use machine learning to sell iPhones to people that have reviewed your Apple product articles.
Dynamic pricing
This one is a good area for implementing machine learning. Instead of offering a fixed price at all times, you could tie pricing to time of the day or a type of user. A good example here is Uber. Its algorithm analyses a destination a user wants to go to and nearby routes to offer a special price.
Customer service
Machine learning and customer service are a natural fit. Lots of companies have already improved their services using chatbots to address customer requests in no time.
There’s no denying it, chatbots boost sales. That can be backed up by the case of Sephora, who increased their sales up to 11% after integrating a chatbot assistant into their Facebook page. The secret is to create a chatbot that would capture your brand voice while providing a service akin to a human assistant.
What to consider when implementing machine learning?
According to EmerJ blog, several factors warrant the usage of machine learning at an organization.
Recent and accurate data
Clean data is the cornerstone of any data science effort. But it also needs to be up to date, which is particularly true for the rapidly changing fields. If your data is not recent, it can hardly present any predictive value. From years of operating, you probably have gained a large volume of data. It needs to undergo the cleansing process before your data science team can use it.
Labeled datasets
Unsupervised learning can be applied in many cases of unlabeled data, but it’s not the best decision to start out with unsupervised ML. More profit can be gained from old labeled datasets.
Facebook owns a vast amount of labeled data like users’ faces from various angles, in different poses and with different expressions. The company uses this data is used to train algorithms, making them highly accurate. Another case here is Google that analyzes the relevance of search results based on the rates of clicks, the usability of pages, user scroll depth, and other factors. There cannot be a set of clear rules for showing the right results with such a number of factors at play. Supervised datasets are also vital to credit companies. While there’s a huge amount of transactions, it’s possible to track connections in purchases, locations tied back to individual users. Thus, it’s possible to predict fraudulent actions. Machine learning can also streamline customer support by identifying and marking types of requests received via emails or phone, such as technical problems, refunds, shipping problems, and more.
Room for error
As suggested by EmerJ, machine learning cannot be applied in an environment where errors are intolerable. After all, machine learning is a malleable, adaptive skill that continues to grow as the company endures. An example of such is a system that recognizes an amount or a company name on an invoice and then pays it. An error in such a case results in overpaying the amount or paying the wrong company. In this example, it’s best to apply machine learning to sort types of bills, but the payment part requires human involvement.
Who is ahead of the curve in the business machine learning?
The company has long since implementing ML algorithms to improve user experience. Pinterest uses image search and recommendation scripts developed by Kosei, a machine learning company. Additionally, the social medium leverages various algorithms to filter content as well as for monetization purposes.
The social media giant uses machine learning to take your communication with chatbots to the next level, making it more human-like. There are also algorithms for filtering out spam and irrelevant content.
IBM
A global giant named IBM is also mastering machine learning. The most prominent project is IBM Watson used in healthcare.
The company uses algorithms to organize the news feed so that users can see the most relevant content. Analyzing tweets and likes of each user helps to achieve that.
Through machine learning, the behemoth improves search results and page ranking and boosts speech recognition and translation speed.
Baidu
Baidu, a Chinese search engine, strives to keep pace with Google, investing heavily in machine learning. One of the most significant developments based on machine learning algorithms is Deep Voice, a human voice technology capable of generating such natural voices that are barely distinguishable from natural ones. The technology can even “clon” voices, pronunciation, stress, timbre, and pitch of sounds in spoken language.
Read also: CTO Guide To The Business of Cloud Computing
Afterword
If you see the benefit of using machine learning to improve your business, it’s useful to consider a few things. Starting out with machine learning doesn’t necessarily take an in-house team of specialized experts. Algorithms can be created by service providers or software developers with knowledge of data science libraries. What you can do on your part is to ensure that the data is recent and accurate. Think about tasks that can be automated to increase speed and scope – that area can be improved by machine learning. Above all, consider that machine learning isn’t there to put humans out of work. Instead, it can be used to reduce routine work, boost speed and improve accuracy.
Our machine learning and data science specialists from NCube support the data efforts of FRST, a Blockchain analytics and strategy company. We can set up a team of skilled data science specialists and machine learning engineers from Ukraine for your project. Let’s talk?
Recommended articles